Autonomous deployment in infrastructure-free environments
Autonomous deployment of fleets of robots responds to a key feature that is crucial for making autonomous transportation systems economical. This will be a step-changing feature, as it will drastically curtail deployment time and costs. Not only the involvement of deployment engineers will be significantly reduced, but additional infrastructure in the environment will be curtailed. This objective goes beyond merely automatically building an environment map for loalisation, and includes also automatic semantic labelling, and automatic verification of the map. The deployment process will be autonomous and it will continue over the life-span of the system: modifications to the environment (e.g., changing buffer zones for pallets, changing movement patterns of other agents, or even relocation of the warehouse shelves), different operational conditions (e.g., external deadlines and precedences to be met), and changes in the fleet (e.g., adding or removing robots, or detecting that the sensors for a particular truck are drifting) will be automatically detected and accounted for.
Long-term operation
Reliable long-term operation is an essential feature of any industrial transportation system. To this end, ILIAD will develop novel techniques for monitoring and maintaining the knowledge about the environment acquired during autonomous deployment, e.g., for a particular logistics warehouse. By monitoring typical human activity and the effects of those actions on the environment over time, the system will be able to adapt its representations in a proactive way by taking actions to resolve the uncertainty and to correct errors. This will result also in up-to-date maps and successful localisation despite major changes to the environment over time.
On-line, self-optimising fleet management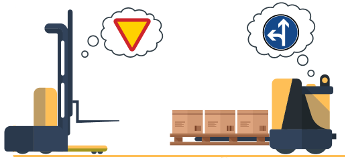
ILIAD specifically targets the development of a planning and coordination system for fleets of industrial vehicles. The core elements of a holistic and flexible approach to fleet management are (1) task allocation, (2) motion planning, (3) coordination, and (4) their integration into a closed loop with distributed vehicle execution. Only a partial integration of pairs of these sub-problems has been achieved in the scientific community. The lack of an integrated solution is directly responsible for inefficiencies in fleet deployment and sub-optimal, inflexible, ad-hoc solutions. ILIAD will address the integration of sub-problems (1–3). The result will allow to progress w.r.t. current practice in industrial fleet automation, in which coordination and task allocation are largely performed off-line, task allocation does not consider sophisticated optimisation criteria, vehicle paths are hand-crafted, vehicle motions are subject to manually synthesised traffic rules, and the consequences of execution time trajectory adjustments of one vehicle are not reflected on the behaviours of other vehicles. ILIAD will also study the combination of centralized approaches with distributed algorithms for motion coordination and task assignment in case of a large number of vehicles and limited communication bandwidth. Scenario characteristics, mission requirements and available resources will be key factors on which a balanced level of decentralisation/centralisation in the coordination system will be determined.
Human-aware AGV fleets in shared environments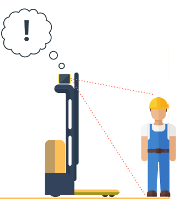
Typical interaction strategies between most current AGVs and human workers are simple: the vehicle stops whenever an obstacle, human or not, is in front of it. This is safe yet highly inefficient and attempts to improve on this are bound by the trade off between safety and task-efficiency. In ILIAD, we argue that robots with the capacity to distinguish humans from other obstacles, learn models of their behavior and plan with accurate predictions from those models will make them more task-efficient and at the same time more safe and trustworthy to surrounding humans. To this end, we plan to develop methods, theoryand experiments to recognise and analyze humans, learn human behavior models over short and long time scales and using the models to generate legible and socially normative robot motion. The result are robots with a deeper understanding of social context and how context is likely to unfold during the interaction. In this way we optimize the safety–efficiency trade-off, facilitating safe yet significantly more efficient AGV operation in mixed man-machine environments.
Unpacking and palletising of goods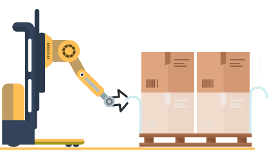
Another objective of ILIAD is the development of underactuated and compliant end effectors for the manipulation of goods. In particular, pallets will be unwrapped and good will be localised, recognised and picked by the ILIAD end effector. Finally, goods will be positioned on a second pallet ready for delivery. The motion of the manipulator will be based on information about the goods and the environment from the perception system. The goal is to avoid undesirable contacts with the environment and to optimise the positions of the boxes on the new pallet.
Human safety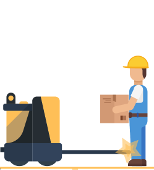
In order for the technologies developed in ILIAD to become practically useful and exploitable, it is imperative that the system can run both safely and efficiently. Certifying the safety of a robotic system that works alongside humans is difficult in general, and especially so when key components include perception or learning. Therefore, in ILIAD we go beyond merely using safety certified sensors that stop the system when collision risks are detected, and instead address the bigger issue of undertaking a systematic study of human safety in shared environments. We will build a large injury safety database, containing the basic human injury biomechanics during collisions, and connect it to safe motion control and planning for preventing human injury. Based on the results, we elaborate safety recommendations for standardisation committees, thus setting the stage for future safety certification and validation that will include, but not be limited to, fleets of advanced AGVs with manipulation capabilities in mixed environments, facilitating the transition to automation.